The Surprisingly Critical (Yet Often Undervalued) Ingredient For AI Success
by Mark Marinelli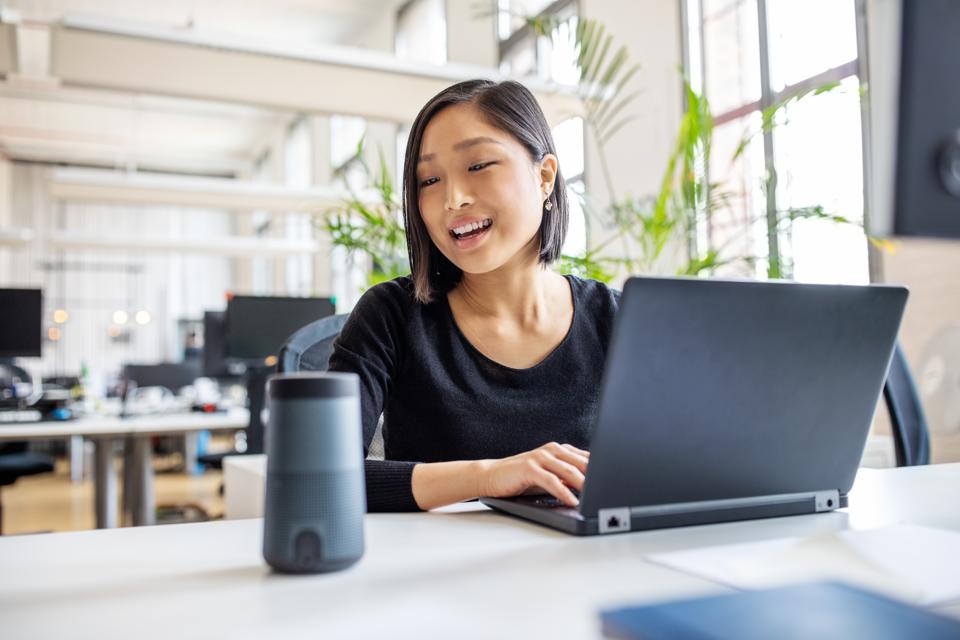
Most organizations experience some failures when it comes to their AI projects — in fact, a quarter of those surveyed by IDC reported up to a 50% failure rate.
This is disappointing for companies trying to adopt AI. They figure out the problem they want to solve, start gathering the needed data and may even figure out a solid data mastering plan. Then, even after they conduct a successful proof of concept and demonstrate how the use of AI will improve the business, things stall out, and frustrations rise.
Not surprisingly, lack of the right data and lack of available skills top the list of problems. But even if these issues are solved, projects often still fall short. So, what can make AI efforts more likely to pay off?
The Missing Link: The Human-Machine Interface
The solution is often overlooked. AI is artificial intelligence, and ML is machine learning. And both of these need to be taught how to function properly by the actual human beings who know the most about the data. Throughout the process of building and maintaining a useful model, it’s important to make sure you’re working closely with subject matter experts who are directly impacted by the quality and accuracy of results and who can provide the most useful feedback.
Companies often make the mistake of assuming that Ph.D.s who know a lot about probabilistic math can come in and figure everything out. Unfortunately, that’s almost always a recipe for failure. They understand how to work with data structurally, but they are disconnected from the implications of the outputs they produce and from the business context of how the data was collected.
For example, adopting a fully automated data cleaning model where machines work on the data without an expert’s approval is likely to lead to disappointing outcomes. Also, requiring data users to dedicate long sessions to training ML models or asking them repetitive questions about the data will discourage essential input from those who can help the most. The question is: How can we get the most leverage out of the skills we have in abundance — knowledge about the data — so that our machines are learning the right lessons?
When it comes to ML and AI, active learning is the right model, intelligently soliciting the most impactful feedback from users with minimal effort from them. To enable active learning, what’s needed is an intuitive human-machine interface throughout the workflow. Humans must be involved when the machine needs guidance, approval or verification. It’s crucial to have full synergy between users, experts and machine learning — a continuous but low-touch feedback loop.
Taking A Cue From The Consumer World
The good news is that making ML algorithms better doesn’t have to be an arduous or time-consuming task for business users. Think about how AI models get smarter in the consumer world. Consider the example of OpenTable or Google Reviews in the restaurant space. Diners are asked for curated feedback in the context of their live experience. Does this restaurant offer delivery? Is the food any good? Then, models use the data to provide suggestions for everyone. Consumers probably don’t think of themselves as experts, but they are because they have recent firsthand knowledge and experience.
The enterprise world could greatly benefit from taking a similar scalable, behind-the-scenes approach that acknowledges that subject matter experts are essential to making algorithms consistently better. There needs to be a shift in perception about who needs to contribute to AI projects, and it’s not just data scientists.
Building Trust In Machines
There’s another benefit to involving data citizens in the process where their input is needed: They begin to trust the models because they know what goes into them, and trust is a crucial element in AI success. Only by participating and helping to curate data will people start to feel confident about the decisions machines make.
Always turn to humans to figure out if models are explainable. Why did it reach the output it did? If a model cannot be explained, perhaps it is time to go with a simpler model.
A Final Thought
It’s easy to become enamored with ML and AI technologies and all that they can offer. But it’s important to remember that at the end of the day, it’s often humans who determine the success or failure of the projects using these technologies. While humans cannot match the raw compute power, we uniquely possess the knowledge and critical judgment these systems rely on. In other words, machines need us. Working together, machines and humans can accomplish great things.
Forbes Technology Council is an invitation-only community for world-class CIOs, CTOs and technology executives. Do I qualify?