Moving From Surveillance To Intelligent Actions Using Digital Eyes
by Nallan Sriraman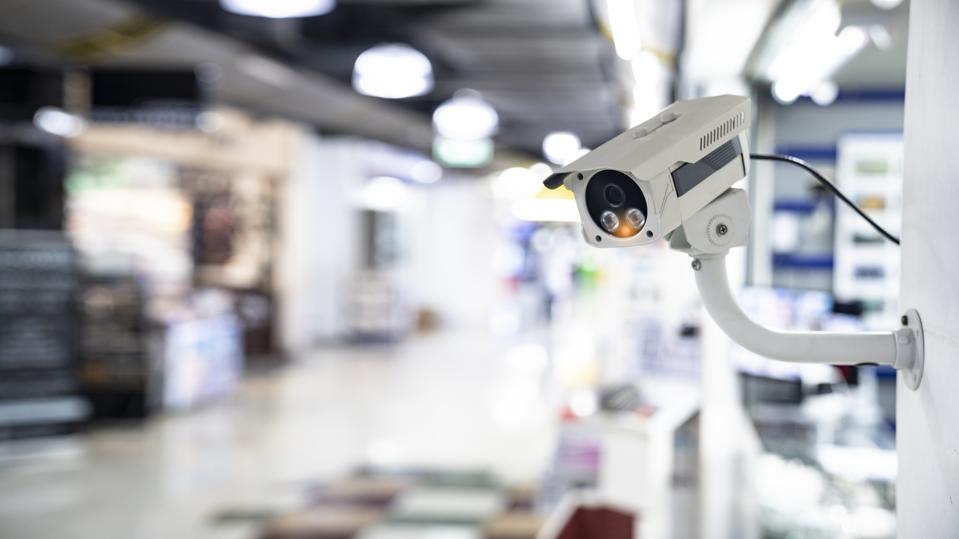
Surveillance cameras are the modern-day digital eyes. They're like Big Brother, the fictional character from George Orwell's dystopian novel Nineteen Eighty-Four, watching over us or watching out for us, depending on your view. But this is changing fast. Artificial intelligence (AI) gives these digital eyes the brains to analyze live video in real time with no human intervention.
The idea is to leverage machine learning (ML) and other AI techniques to automatically recognize temporal and spatial events in a video stream, such as assembly line blockages, suspicious movement in a secure area, and smoke or fire. Companies can use existing surveillance technology along with internet-enabled data collection and cloud-based ML processing for a range of uses and applications to enhance situational awareness.
These technologies aren't new, so why now?
High-risk industry sectors have been effectively using these tools for many years. However, the availability of cost-effective, internet-enabled cameras with complex ML to process the digital stream in real time has made it more efficient and viable than ever. Enterprises should take advantage of these technologies and build next-generation digital video monitoring systems across their sites.
What are some emerging use cases?
Historically, the health and safety industry has invested in video surveillance solutions to ensure staff safety while conducting regulated high-risk activities. Video analytics allows us to understand the data behind visual streams. It can detect incorrect processes executed by a staff member and alert the proper remediation. Slip, trip and fall monitoring is also valuable. It can process images to detect if a person has fallen and alert emergency management staff when needed. Within the current Covid-19 environment, these video streams can identify the proper wearing of face masks, social distancing and hand hygiene measures.
Enterprises can also carry out classic intruder detections more robustly because the algorithms can now filter out motion caused by wind, rain, snow or animals. These systems help collect evidence for investigations and litigations, offering not only visual evidence, but also the underlying inferences.
Additionally, video analytics can help us understand customer behaviors in a store, such as how long they browse an aisle, what they are looking at and what they buy or don't buy. Video analytics, combined with augmented reality, can help enhance the consumer experience with fashion and beauty by providing immersive, real-life experience before buying the products.
Many manufacturing facilities already use digital visual inspection for quality management. In a high-speed labeling machine, a camera combined with ML can detect a misaligned label on a pack and reject the main assembly line, or a camera may detect cracked bottles before the fill or a foreign object entering the assembly — the list goes on.
Enterprises can take advantage of these uses cases without having to invest in bespoke cameras and integrate into the broader AI-enabled digital vision ecosystem.
How do they work?
These systems perform real-time monitoring to detect objects, object attributes, movement patterns or behavior related to the environment. We can also analyze historical data to mine insights and trends. Typically, we can analyze video content in two ways: in real time (by triggering alerts for specific events and incidents that unfold in the moment) or after processing. A typical AI-enabled system is composed of two things:
- Digital video stream: The system should integrate with any video source — the key is the angle of the camera.
- Processing (central versus edge): While traditional CCTVs ran the processing locally, an AI-enabled system can embed the processing in the cameras themselves, a strategy known as edge processing. In recent years, with processing costs coming down, we've seen several cameras with real-time processing capabilities. The industry best practice is, whenever possible, to go with real-time processing on the cameras and the forensic analysis functionalities centrally in the cloud.
What are the challenges?
Traditional CCTV architecture may not be suitable for these use cases and could require upgrades to cameras, networks such as Wi-Fi or 5G, and increased storage and processing needs.
Another challenge is the existing camera angles. For example, leveraging this capability to monitor social distancing during Covid-19 will require streams from at least two cameras with right angles to measure the distance accurately. We have seen significant false-positive alerting while using only one camera for this use case. Depending on the use, we may require additional, special-purpose cameras with right angles that differ greatly from existing CCTV surveillance cameras and their angles.
What are the implications for enterprises?
There are multifaceted social and legal issues surrounding AI-enabled video analytics. While there is no unified legislation on how to use these techniques across the globe, responsible enterprises must declare their intent to use these technologies and make sure their staff is fully supportive of these capabilities. They must ensure full adherence to privacy regulations in various countries and fully embrace their own AI ethics while building them.
Conclusion
AI-enabled video analytics, portrayed in this article as "digital vision," can offer significant value to enterprises today, even with the challenges and implications. But it's crucial that enterprises that embrace these technologies do so while fully adhering to local privacy regulations and their own ethical standards. This can enable them to improve decisions rather than base them on circumstantial or subjective opinions.
As firms look at the future of the workplace after Covid-19, they may want to consider if and how AI-enabled digital vision technologies can improve their employees' experience in their work environment.
Forbes Technology Council is an invitation-only community for world-class CIOs, CTOs and technology executives. Do I qualify?